
Face reconstruction and tracking is a building block of numerous applications in AR/VR, human-machine interaction, as well as medical applications. Most of these applications rely on a metrically correct prediction of the shape, especially, when the reconstructed subject is put into a metrical context (i.e., when there is a reference object of known size).
A metrical reconstruction is also needed for any application that measures distances and dimensions of the subject (e.g., to virtually fit a glasses frame). State-of-the-art methods for face reconstruction from a single image are trained on large 2D image datasets in a self-supervised fashion. However, due to the nature of a perspective projection they are not able to reconstruct the actual face dimensions, and even predicting the average human face outperforms some of these methods in a metrical sense.
To learn the actual shape of a face, we argue for a supervised training scheme. Since there exists no large-scale 3D dataset for this task, we annotated and unified small- and medium-scale databases. The resulting unified dataset contains about 2300 identities with corresponding images. We made this dataset publicly available.
To this end, we take advantage of a face recognition network pretrained on a large-scale 2D image dataset, which provides distinct features for different faces and is robust to expression, illumination, and camera changes. Using these features, we train our face shape estimator in a supervised fashion, inheriting the robustness and generalization of the face recognition network.
Our method, which we call MICA (MetrIC fAce), outperforms the state-of-the-art reconstruction methods by a large margin, both on current non-metric benchmarks as well as on our metric (15% and 24% lower average error on NoW, respectively).
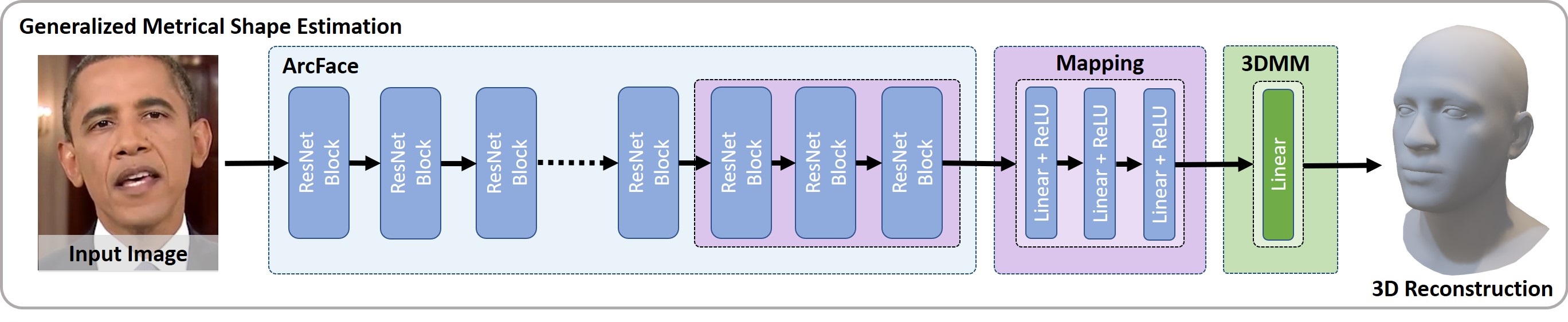
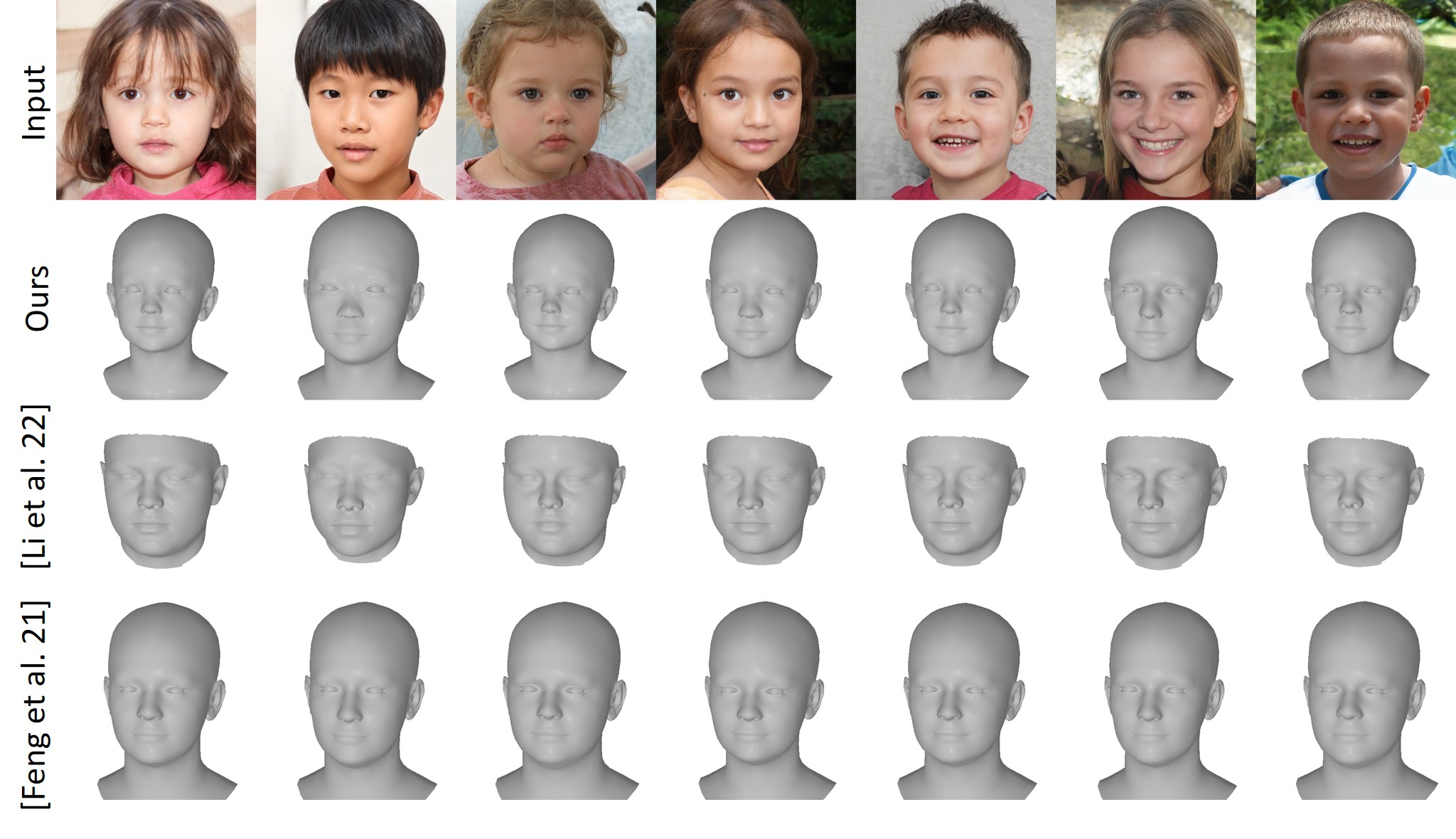
Video
BibTeX
@inproceedings{zielonka2022mica,
title = {Towards Metrical Reconstruction of Human Faces},
author = {Wojciech Zielonka and Timo Bolkart and Justus Thies},
booktitle = {ECCV},
year = {2022}
}