We present SynShot, a novel method for the few-shot inversion of a drivable head avatar based on a synthetic prior. We tackle two major challenges. First, state-of-the-art monocular avatar models struggle to generalize to new views and expressions, lacking a strong prior and often overfitting to a specific viewpoint distribution. Second, training a controllable 3D generative network requires a large number of diverse sequences, for which pairs of images and high-quality tracked meshes are not always available. Moreover, under General Data Protection Regulation, the storage and use of real datasets are highly regulated. Users are often required to delete trained models and remove any derivatives of the real data within a specified time frame if a subject from the dataset withdraws their consent, as guaranteed by law. This process is very cumbersome from a data management perspective, whereas synthetic datasets are not subject to these regulations.
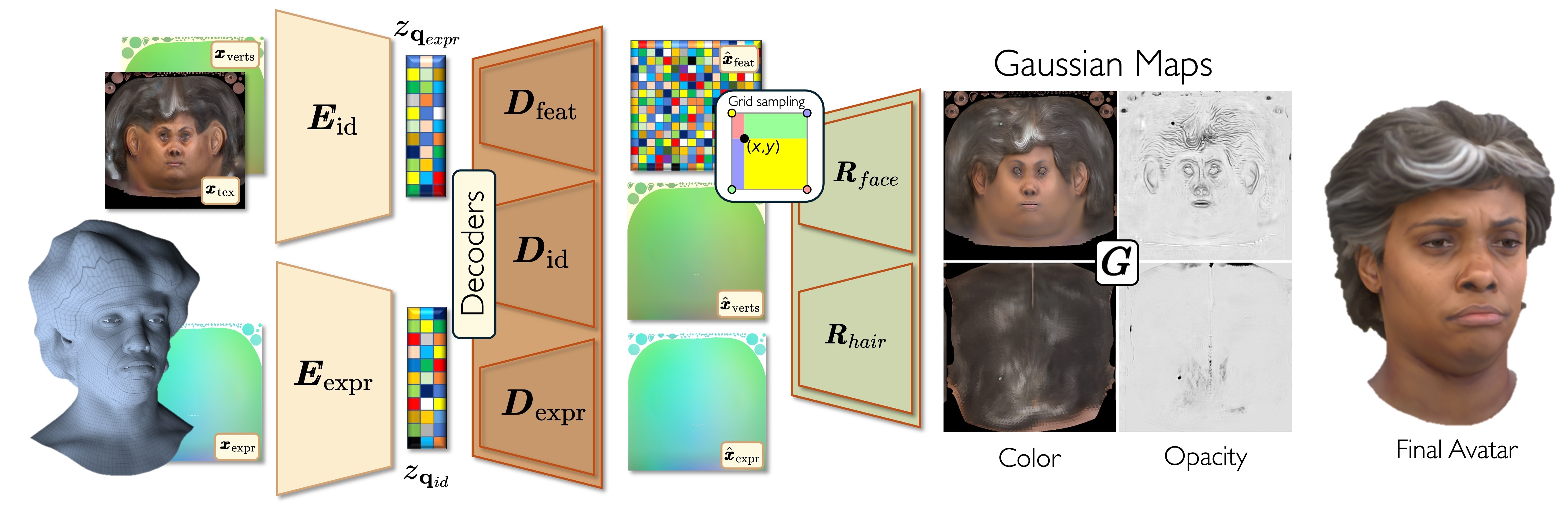

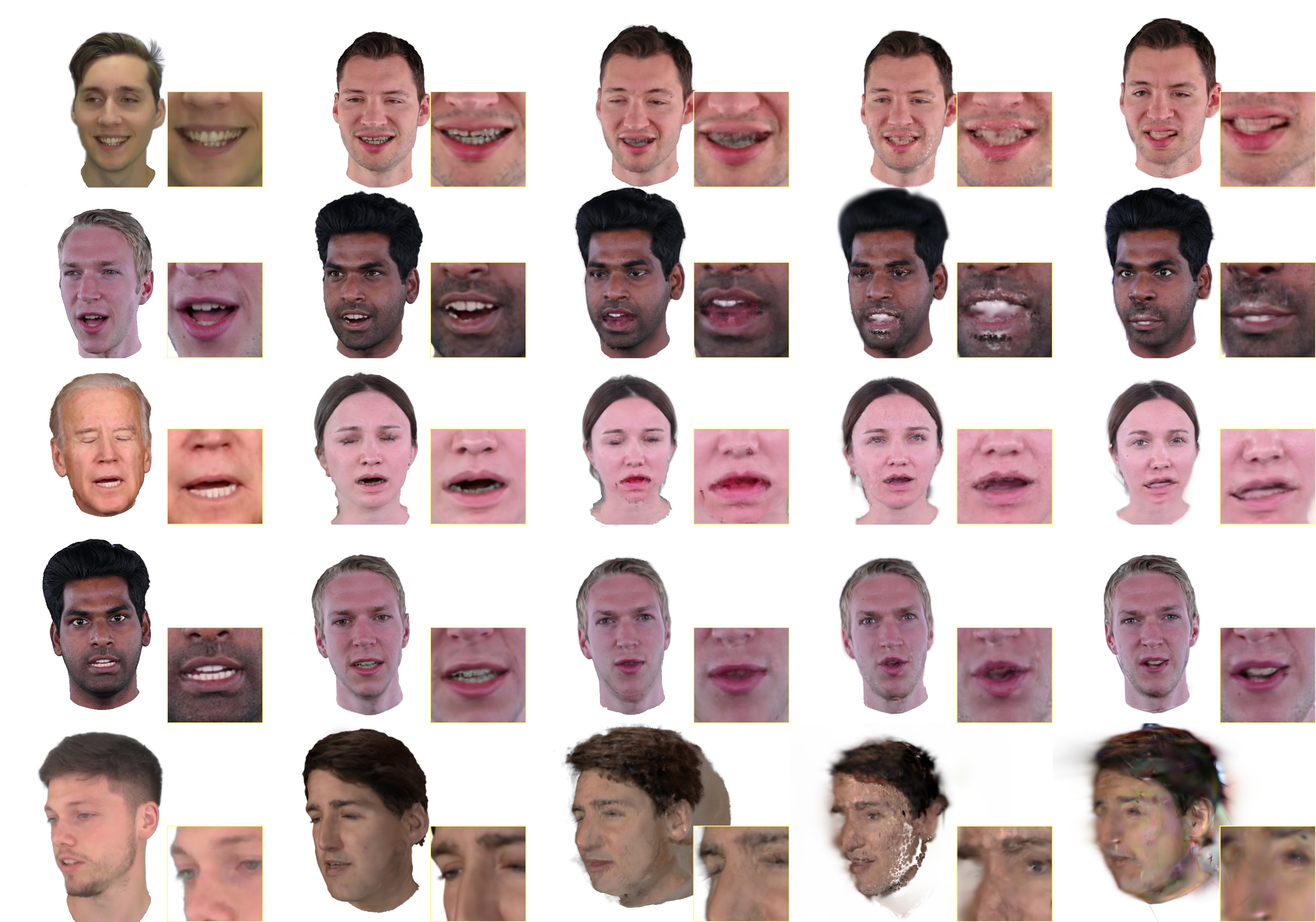
Video
BibTeX
@inproceedings{zielonka2025synshot,
title = {Synthetic Prior for Few-Shot Drivable Head Avatar Inversion},
author = {Wojciech Zielonka and Stephan J. Garbin and Alexandros Lattas and George Kopanas and Paulo Gotardo and Thabo Beeler and Justus Thies and Timo Bolkart},
booktitle = {CVPR},
month = {June},
year = {2025},
}