We present personalized Gaussian Eigen Models (GEMs) for human heads, a novel method that compresses dynamic 3D Gaussians into a low-dimensional linear space. Our approach is inspired by the seminal work of Blanz and Vetter, where a mesh-based 3D morphable model (3DMM) is constructed from registered meshes. Based on dynamic 3D Gaussians, we create a lower-dimensional representation of primitives which is applicable to most 3DGS head avatars. Specifically, we propose a method to distill the appearance of a mesh-controlled UNet Gaussian Avatar using a ensemble of linear eigenbasis. We replace heavy CNN-based architectures with a single linear layer improving speed and enabling a range of real-time downstream applications. To create a particular facial expression, one simply needs to perform a dot product between the eigen coefficients and the distilled basis. This efficient method removes the requirement for an input mesh during testing, enhancing simplicity and speed in expression generation. This process is highly efficient and supports real-time rendering on everyday devices, leveraging the effectiveness of standard Gaussian Splatting. In addition, we demonstrate how the GEM can be controlled using a ResNet-based regression architecture. Self-reenactment and cross-person reenactment are shown and compared to state-of-the-art 3D avatar methods presenting higher quality and better control. A real-time demo showcases the applicability of the GEM representation.
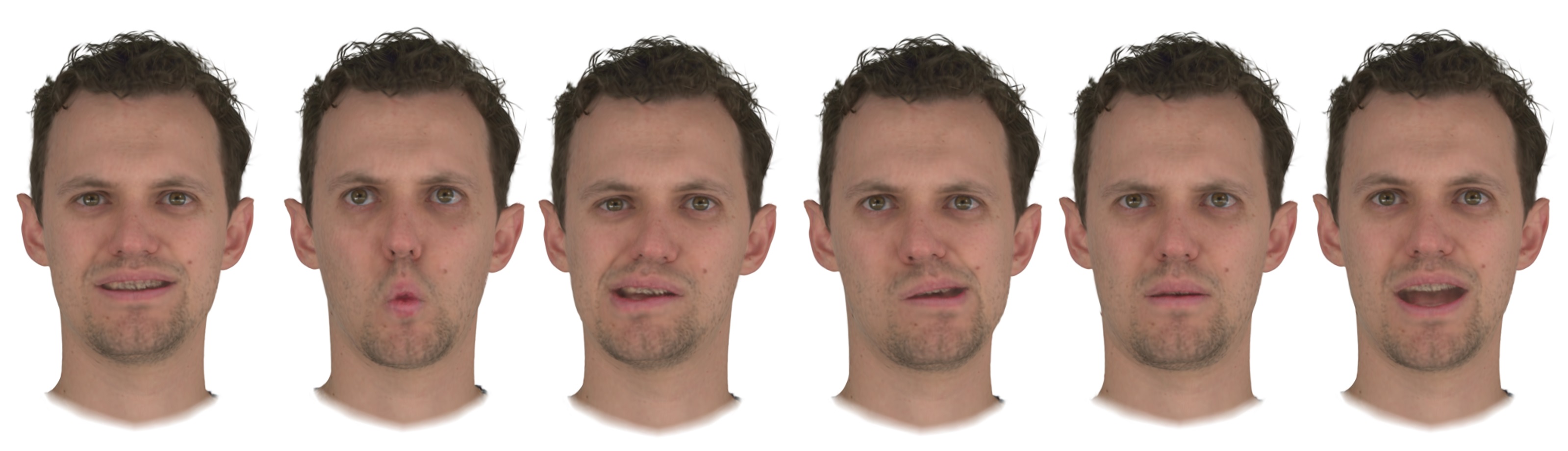
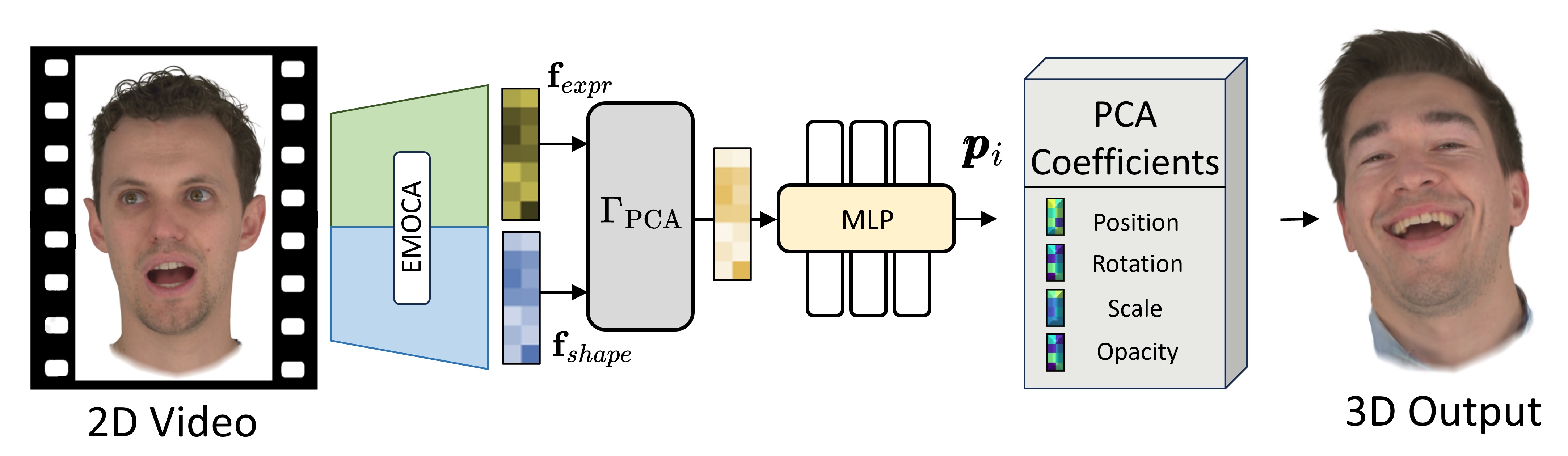
Video
BibTeX
@article{Zielonka2024GEM,
title={Gaussian Eigen Models for Human Heads},
author={Wojciech Zielonka and Timo Bolkart and Thabo Beeler and Justus Thies},
year={2024},
eprint={},
archivePrefix={arXiv},
primaryClass={cs.CV}
}